Methods for Compositional Data using INLA
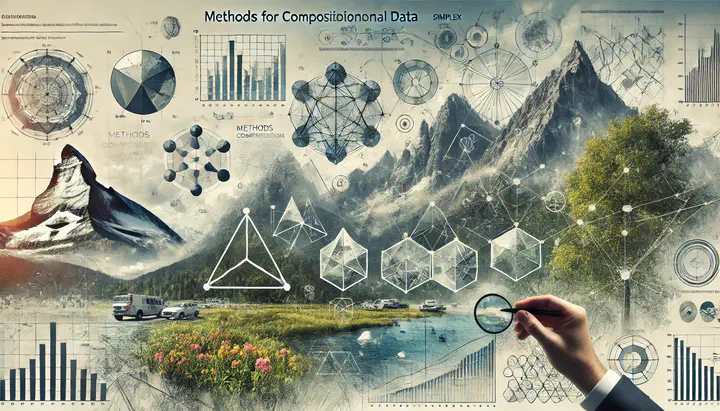
This project centers on advancing Bayesian hierarchical models to analyze compositional datasets, which are prevalent in ecological and environmental studies. The research introduces novel methodologies, such as Dirichlet regression and logistic-normal models, to handle the complexities of compositional data. These methods facilitate better inference and prediction capabilities, addressing challenges like spatial and temporal correlations. The project's applications span across diverse areas, including species distribution modeling and air pollution studies, contributing to enhanced decision-making and policy development in environmental science.